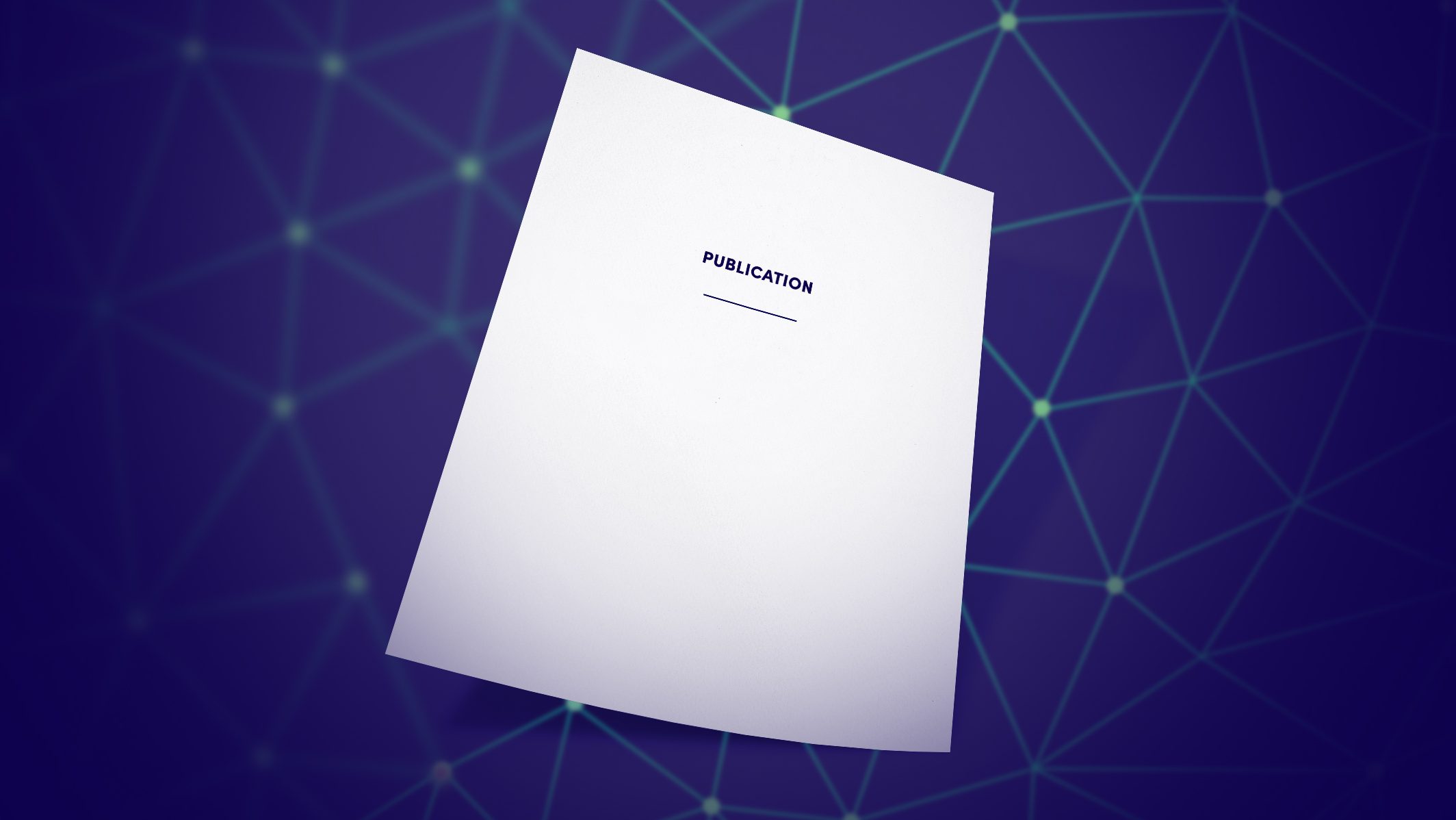
The number of intrusions and attacks against data networks and networked systems increases constantly, while encryption has made it more difficult to inspect network traffic and classify it as malicious. In this paper, an anomaly-based intrusion detection system using Haar wavelet transforms in combination with an adversarial autoencoder was developed for detecting malicious TLS-encrypted Internet traffic. Data containing legitimate, as well as advanced malicious traffic was collected from a large-scale cyber exercise and used in the analysis. Based on the findings and domain expertise, a set of features for distinguishing modern malware from packet timing analysis were chosen and evaluated. Performance of the adversarial autoencoder was compared with a traditional autoencoder. The results indicate that the adversarial model performs better than the traditional autoencoder. In addition, a machine learning pipeline capable of analyzing traffic in near real time was developed for data analysis.
Authors
Samir Puuska, Tero Kokkonen, Janne Alatalo, Eppu Heilimo
Cite as
Puuska S., Kokkonen T., Alatalo J., Heilimo E. (2019) Anomaly-Based Network Intrusion Detection Using Wavelets and Adversarial Autoencoders. In: Lanet JL., Toma C. (eds) Innovative Security Solutions for Information Technology and Communications. SECITC 2018. Lecture Notes in Computer Science, vol 11359. Springer, Cham