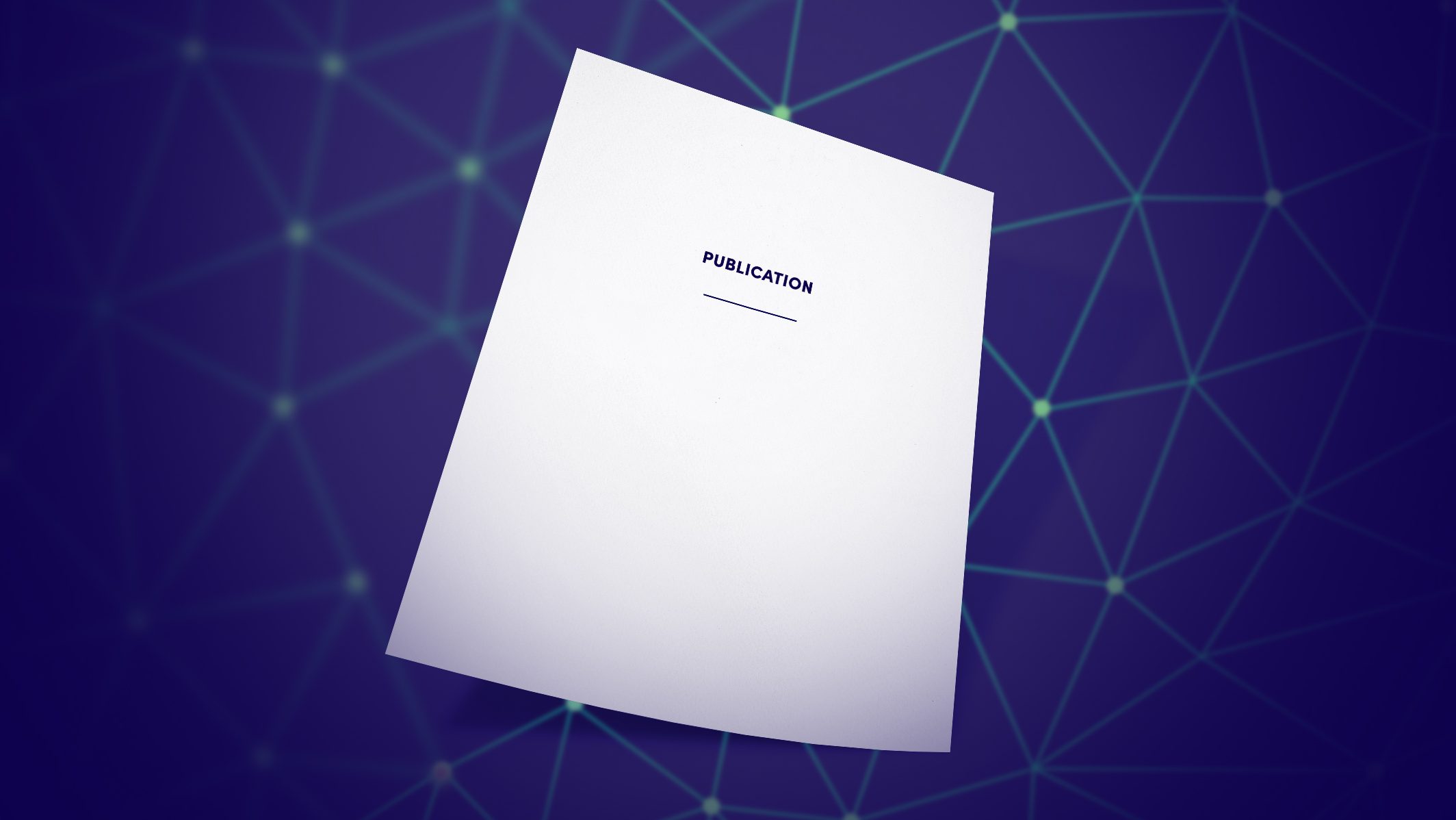
Automatic medical image diagnosis tools are vulnerable to modern model fooling technologies. Because medical imaging is a way of determining the health status of a person, the threats could have grave consequences. These threats are not only dangerous to the individual but also threaten the patients’ trust in modern diagnosis methods and in the healthcare sector as a whole. As recent diagnosis tools are based on artificial intelligence and machine learning, they can be exploited using attack technologies such as image perturbations, adversarial patches, adversarial images, one-pixel attacks, and training process tampering. These methods take advantage of the non-robust nature of many machine learning models created to solve medical imaging classification problems, such as determining the probability of cancerous cell growth in tissue samples. In this study, we review the current state of these attacks and discuss their effect on medical imaging. By comparing the known attack methods and their use against medical imaging, we conclude with an evaluation of their potential use against medical imaging.
Authors
Tuomo Sipola, Tero Kokkonen, Mika Karjalainen
Cite as
Sipola, T., Kokkonen, T., Karjalainen, M. (2023). Model Fooling Threats Against Medical Imaging. In: Sipola, T., Kokkonen, T., Karjalainen, M. (eds) Artificial Intelligence and Cybersecurity. Springer, Cham. https://doi.org/10.1007/978-3-031-15030-2_13
Publication
https://dx.doi.org/10.1007/978-3-031-15030-2_13
Acknowledgements
This research is partially funded by The Regional Council of Central Finland/Council of Tampere Region and European Regional Development Fund as part of the Health Care Cyber Range (HCCR) project and The Cyber Security Network of Competence Centres for Europe (CyberSec4Europe) project of the Horizon 2020 SU-ICT-03-2018 program. The authors would like to thank Ms. Tuula Kotikoski for proofreading the manuscript.