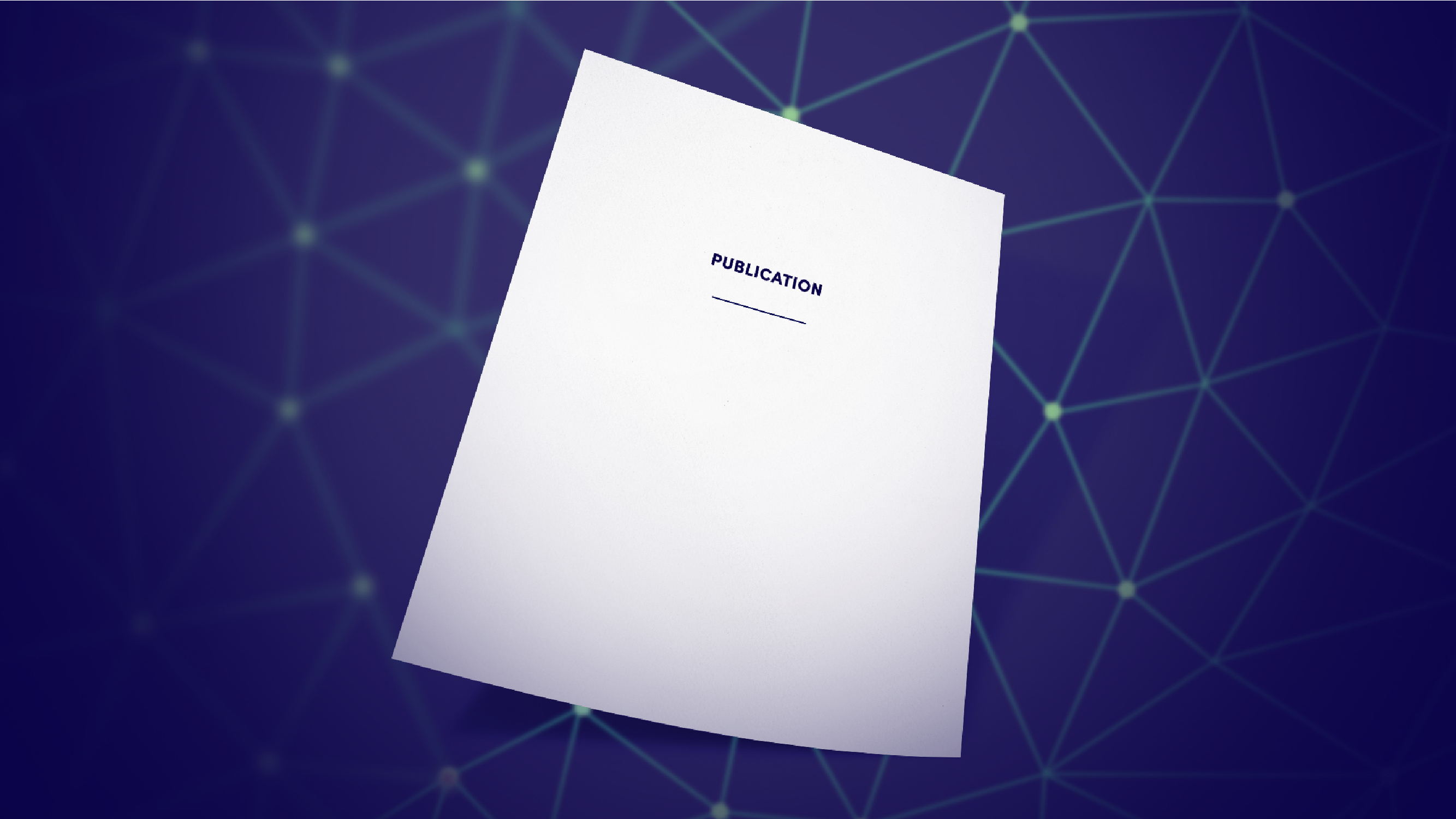
Satellite-based synthetic aperture radar (SAR) images can be used as a source of remote sensed imagery regardless of cloud cover and day–night cycle. However, the speckle noise and varying image acquisition conditions pose a challenge for change detection classifiers. This article proposes a new method of improving SAR image processing to produce higher quality difference images (DIs) for the classification algorithms. The method is built on a neural network-based mapping transformation function that produces artificial SAR images from a location in the requested acquisition conditions. The inputs for the model are: previous SAR images from the location, imaging angle information from the SAR images, digital elevation model, and weather conditions. The method was tested with data from a location in North-East Finland by using Sentinel-1 SAR images from the European Space Agency (ESA), weather data from Finnish Meteorological Institute (FMI), and a digital elevation model from National Land Survey of Finland (NLS). In order to verify the method, changes to the SAR images were simulated, and the performance of the proposed method was measured using experimentation where it gave substantial improvements to performance when compared to a more conventional method of creating DIs.
Authors
Janne Alatalo, Tuomo Sipola, and Mika Rantonen
Cite as
J. Alatalo, T. Sipola and M. Rantonen, “Improved Difference Images for Change Detection Classifiers in SAR Imagery Using Deep Learning,” in IEEE Transactions on Geoscience and Remote Sensin, vol. 61, pp. 1-14, 2023, Art no. 5218714, doi: 10.1109/TGRS.2023.3324994.
Publication
Article: https://doi.org/10.1109/TGRS.2023.3324994
Data: https://doi.org/10.23729/7b22c271-5e25-40fe-aa6a-f5d0330b1872
Code: https://github.com/janne-alatalo/sar-change-detection
Acknowledgements
This research was conducted at the Institute of Information Technology of Jamk University of Applied Sciences as part of Data for Utilisation – Leveraging digitalisation through modern artificial intelligence solutions and cybersecurity project, coADDVA – ADDing VAlue by Computing in Manufacturing project, and Finnish Future Farm project. This work uses modified Copernicus Sentinel data 2020-2021. The authors thank Eppu Heilimo for feedback on the draft manuscript. They wish to acknowledge CSC – IT Center for Science, Finland, for hosting the dataset