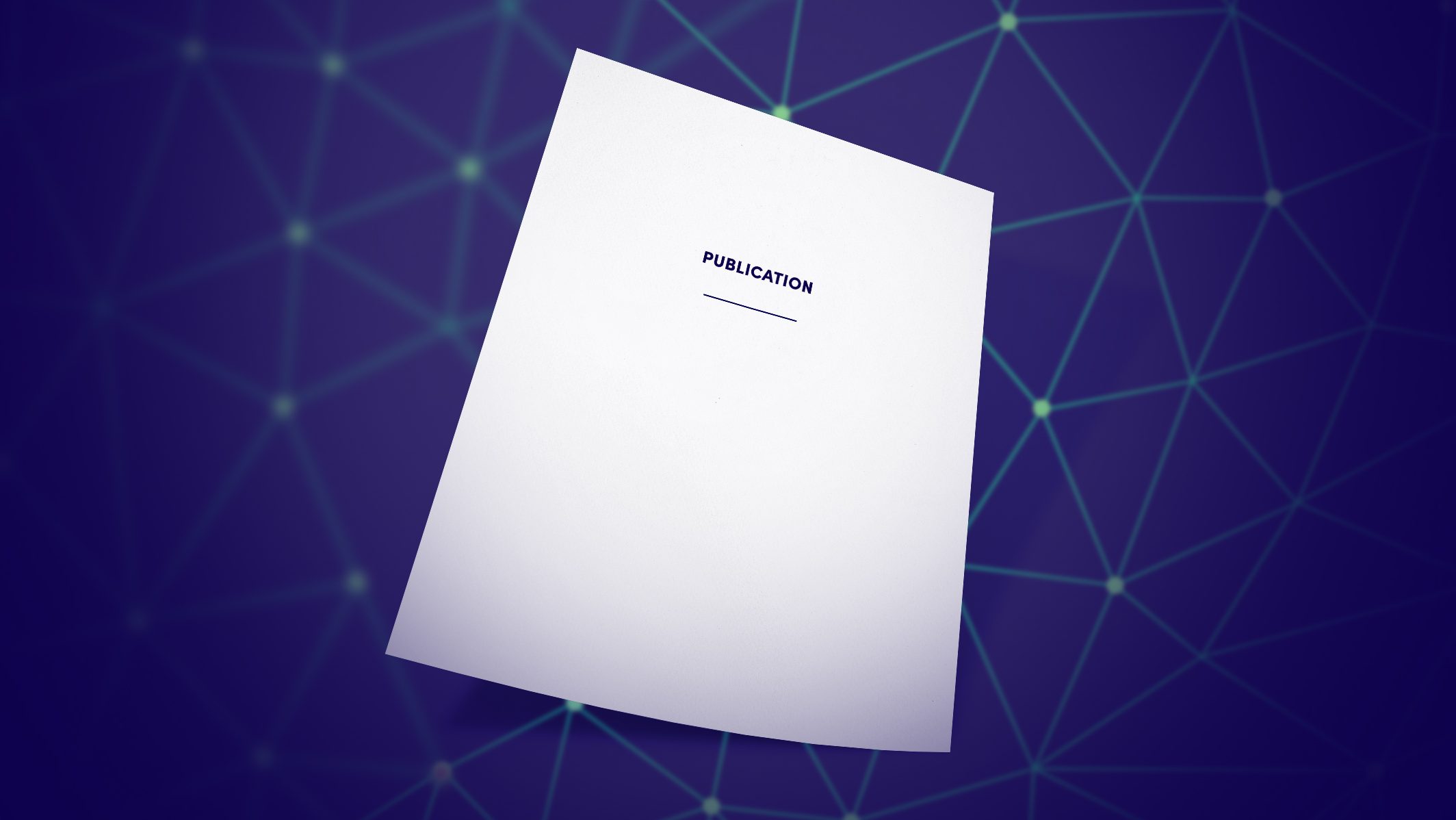
In the field of medical imaging, artificial intelligence solutions are used for diagnosis, prediction and treatment processes. Such solutions are vulnerable to cyberattacks, especially adversarial attacks targeted at machine learning algorithms. One-pixel attack is an adversarial method against image classification algorithms based on neural networks. In this study, we show that a variational autoencoder can be used to detect such attacks in the context of medical imaging. We use adversarial one-pixel images generated from the TUPAC16 dataset and apply the variational autoencoder as a filter before letting the images pass to the classifier. The results indicate that the variational autoencoder model efficiently detects one-pixel attacks.
Authors
Janne Alatalo, Tuomo Sipola, Tero Kokkonen
Cite as
Alatalo, J., Sipola, T., Kokkonen, T. (2022). Detecting One-Pixel Attacks Using Variational Autoencoders. In: Rocha, A., Adeli, H., Dzemyda, G., Moreira, F. (eds) Information Systems and Technologies. WorldCIST 2022. Lecture Notes in Networks and Systems, vol 468. Springer, Cham.
Publication
https://doi.org/10.1007/978-3-031-04826-5_60
Acknowledgements
This research was partially funded by Cyber Security Network of Competence Centres for Europe (CyberSec4Europe) project of the Horizon 2020 SU-ICT-03-2018 program.
The authors would like to thank Ms. Tuula Kotikoski for proofreading the manuscript.