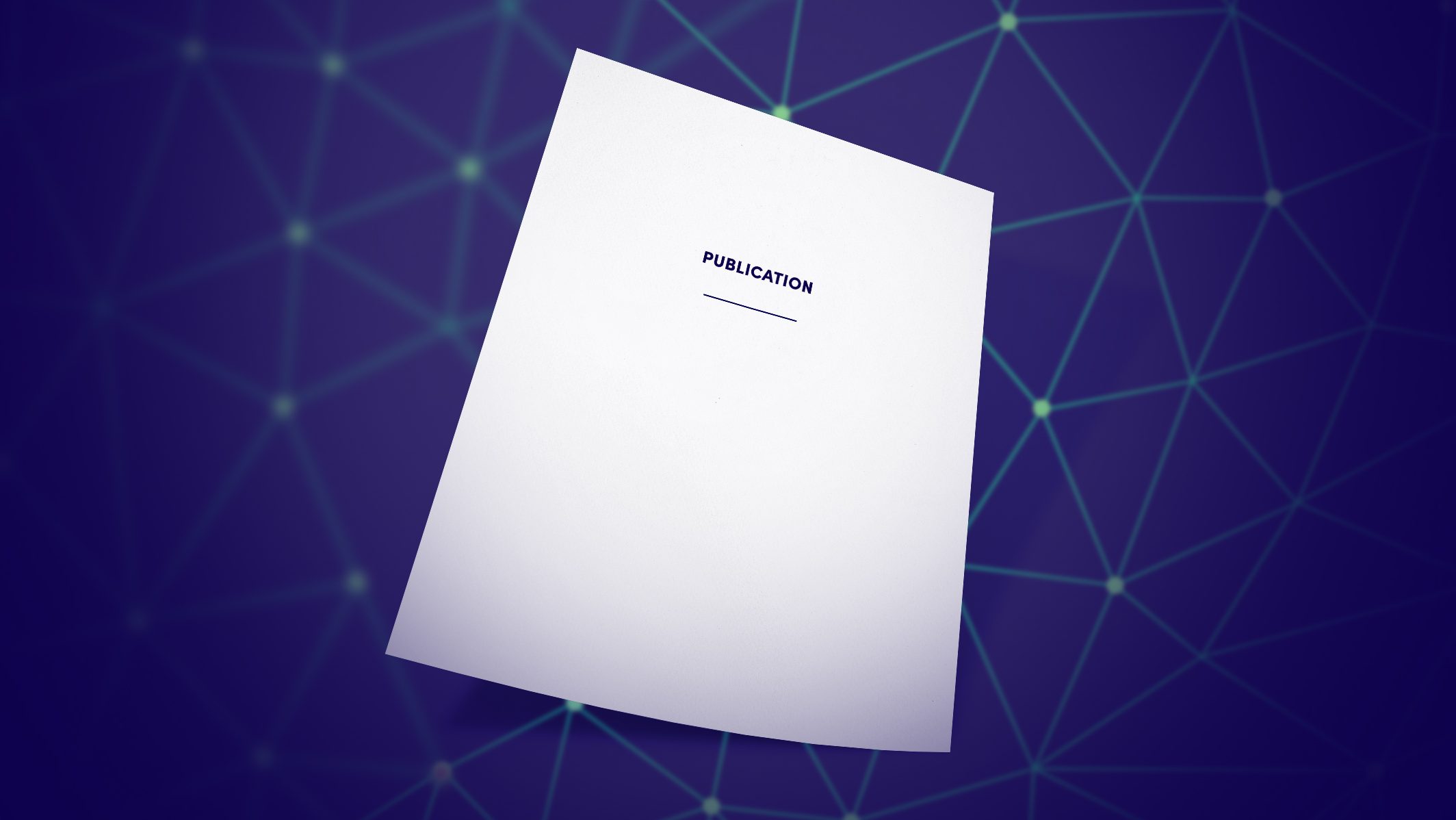
Nowadays, zero-day Denial-of-Service (DoS) attacks become frighteningly common in high-speed networks due to constantly increasing number of vulnerabilities. Moreover, these attacks become more sophisticated, and, therefore, they are hard to detect before they damage several networks and hosts. Due to these reasons, real-time monitoring, processing and network anomaly detection must be among key features of a modern DoS prevention system. In this paper, we present a method which allows us to timely detect various denial-of-service attacks against a computer or a network system. We focus on detection of application-layer DoS attacks that utilize encrypted protocols by applying an anomaly-detection-based approach to statistics extracted from network packets. Since network traffic decryption can violate ethical norms and regulations on privacy, the detection scheme proposed analyzes network traffic without its decryption. The scheme includes the analysis of conversations between a web server and its clients, the construction of a model of normal user behavior by dividing these conversations into clusters and the examination of distribution of these conversations among the resulting clusters with the help of the stacked auto-encoder which belongs to a class of deep learning algorithms. Conversations of clients that deviate from those normal patterns are classified as anomalous. The proposed technique is tested on the data obtained with the help of a realistic cyber environment.
Authors
Zolotukhin Mikhail, Hämäläinen Timo, Kokkonen Tero, Siltanen Jarmo
Cite as
M. Zolotukhin, T. Hämäläinen, T. Kokkonen and J. Siltanen, “Increasing web service availability by detecting application-layer DDoS attacks in encrypted traffic,” 2016 23rd International Conference on Telecommunications (ICT), Thessaloniki, 2016, pp. 1-6.